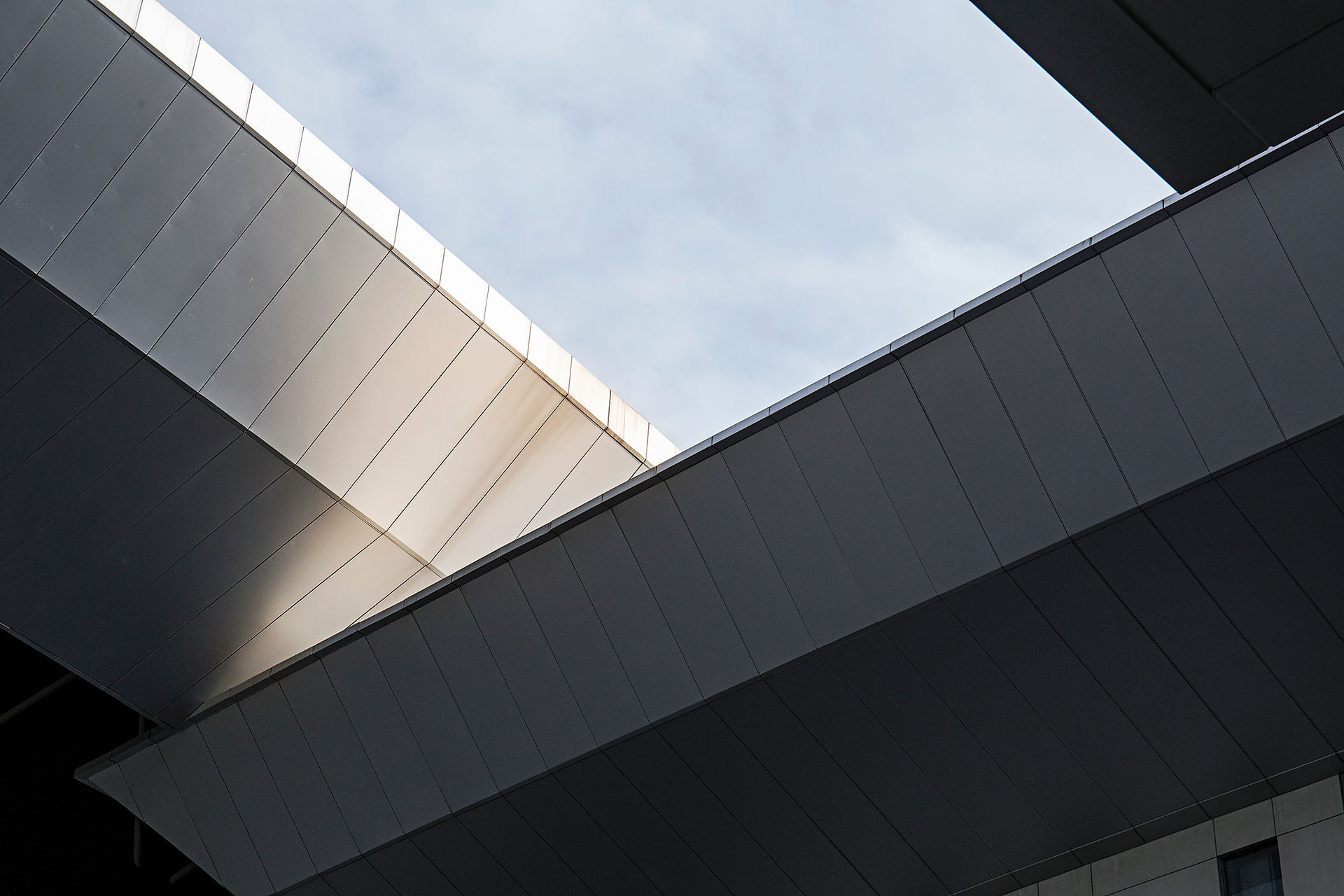
Large-Scale Question Tagging via Joint Question-Topic Embedding Learning

As compared to the traditional community-based question answering sites, like Yahoo! Answers and Wikipedia, which are at a low ebb, social question answering sites, including Quora and Zhihu, are gaining momentum. Besides interactions, the latter enables users to label the questions with topic tags that highlight the key points conveyed in the questions. In this paper, we shed light on automatically annotating a newly posted question with topic tags which are pre-defined and pre-organized into a directed acyclic graph. To accomplish this task, we present an end-to-end deep interactive embedding model to jointly learn the embeddings of questions and topics by projecting them into the same space for similarity measure. In particular, we first learn the embeddings of questions and topic tags by two deep parallel models. Thereinto, we regularize the embeddings of topic tags via fully exploring their hierarchical structure, which is able to alleviate the problem of imbalanced topic distribution. Thereafter, we interact each question embedding with the topic tag matrix, i.e., all the topic tag embeddings. Following that, a sigmoid cross-entropy loss is appended to reward the positive question-topic pairs and penalize the negative ones. To justify our model, we conducted extensive experiments on an unprecedented large-scale social QA dataset obtained from Zhihu.com, and the experimental results demonstrate that our model achieves superior performance to several state-of-the-art baselines.
Copyright (C) <2020> Shandong University
This program is licensed under the GNU General Public License 3.0 (https://www.gnu.org/licenses/gpl-3.0.html). Any derivative work obtained under this license must be licensed under the GNU General Public License as published by the Free Software Foundation, either Version 3 of the License, or (at your option) any later version, if this derivative work is distributed to a third party.
The copyright for the program is owned by Shandong University. For commercial projects that require the ability to distribute the code of this program as part of a program that cannot be distributed under the GNU General Public License, please contact <liqiangnie@gmail.com> to purchase a commercial license.